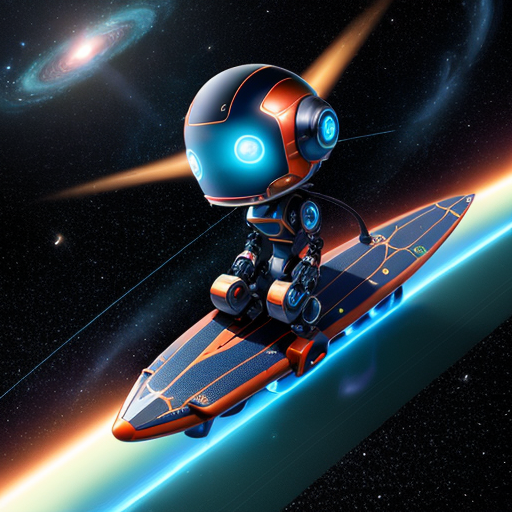
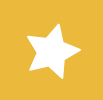
Relevant xkcd video: https://www.youtube.com/watch?v=3EI08o-IGYk
Relevant xkcd video: https://www.youtube.com/watch?v=3EI08o-IGYk
Probably “Trap Adventure 2”.
Imagine an old Mario game where Bowser has the most rediculous traps set up. You need to memorize all of the trap locations as well as have the coordination to tip-toe around them to survive.
Here’s a rough translation to english using Whisper. I’m assuming “rock-climbers” should be “excavators”:
[00:00:00.000 --> 00:00:04.000] Here they are, fortifications are being built all over the Kursk region.
[00:00:04.000 --> 00:00:06.000] The rock-climbers are working.
[00:00:06.000 --> 00:00:09.000] A huge, endless line of anti-tank weapons.
[00:00:09.000 --> 00:00:11.000] There, further, is the Ukrainian.
[00:00:11.000 --> 00:00:13.000] Well, more precisely, the enemy.
[00:00:13.000 --> 00:00:15.000] Because not only the Ukrainian.
[00:00:15.000 --> 00:00:17.000] Here.
I ran the audio through Whisper to translate it. I’d appreciate if someone who understands better could double check it, or explain the reference/saying about hares if they know:
[00:00:00.000 --> 00:00:04.000] Who says that you can’t go abroad?
[00:00:04.000 --> 00:00:09.000] Guys, everyone is going to the army, they are letting us go abroad.
[00:00:09.000 --> 00:00:14.000] Two hares would have gone, but they didn’t.
[00:00:14.000 --> 00:00:17.000] But everything is fine.
[00:00:17.000 --> 00:00:24.000] Here it is, here it is.
[00:00:27.000 --> 00:00:31.000] Not at the border, but in the sky.
[00:00:31.000 --> 00:00:35.000] That’s it, guys, you are abroad.
[00:00:35.000 --> 00:00:38.000] No shit.
[00:00:38.000 --> 00:00:44.000] So if you want, go ahead.
[00:00:44.000 --> 00:00:48.000] - We don’t ask for anything. - We don’t ask for anything.
[00:00:48.000 --> 00:00:51.000] All the guys are happy.
Isn’t the whole point of something like End-to-End Encryption so that not even the company themselves can read your messages?
In that case it wouldn’t matter even if they did turn the info over.
Edit: I read more into the page you linked. Looks like those NSLs can’t even be used to request the contents either way:
Can the FBI obtain content—like e-mails or the content of phone calls—with an NSL?
Not legally. While each type of NSL allows the FBI to obtain a different type of information, that information is limited to records—such as “subscriber information and toll billing records information” from telephone companies.
Found it: https://www.youtube.com/watch?v=45CvTHmt_dI
This is why I prefer it when people specify what kind of “AI” they’re talking about rather than just saying “AI” as a buzzword. I definitely agree with Derbauer here… this is painful.
Great video, he breaks everything down really well…
I might have to start linking to this video rather than trying explain what AI is vs what AI isn’t to those who like to make general statements like “AI is a scam!”.
Getting away from Google Maps has been a tough one. There aren’t many options there, it’s either Google, Apple, Microsoft, or OpenStreetMap.
I’ve been contributing to OSM for my local area as much as possible to update businesses and their opening hours, website, etc., but it’s not a small task.
and more
I bet they included farming equipment in the exemption list…
Ok, first off, I’m a big fan of learning new expressions where they come from and what they mean (how they came about, etc). Could you please explain this one?:
well, you dance and jump over the fire in the bank’s vault.
And back to the original topic:
It isn’t resource efficient, simple as that.
It’s not that simple at all and it all depends on your use case for whatever model you’re talking about:
For example I could spend hours working in Photoshop to create some image that I can use as my Avatar on a website. Or I can take a few minutes generating a bunch of images through Stable Diffusion and then pick out one I like. Not only have I saved time in this task, but I have used less electricity.
In another example I could spend time/electricity to watch a Video over and over again trying to translate what someone said from one language to another, or I could use Whisper to quickly translate and transcribe what was said in a matter of seconds.
On the other hand, there are absolutely use cases where using some ML model is incredibly wasteful. Take, for example, a rain sensor on your car. Now, you could setup some AI model with a camera and computer vision to detect when to turn on your windshield wipers. But why do that when you could use this little sensor that shoots out a small laser against the window and when it detects a difference in the energy that’s normally reflected back it can activate the windshield wipers. The dedicated sensor with a low power laser will use far less energy and be way more efficient for this use case.
Cheers on you if you found where to put it to work as I haven’t and grown irritated over seeing this buzzword everywhere.
Makes sense, so many companies are jumping on this as a buzzword when they really need to stop and think if it’s necessary to implement in the first place. Personally, I have found them great as an assistant for programming code as well as brainstorming ideas or at least for helping to point me in a good direction when I am looking into something new. I treat them as if someone was trying to remember something off the top of their head. Anything coming from an LLM should be double checked and verified before committing to it.
And I absolutely agree with your final paragraph, that’s why I typically use my own local models running on my own hardware for coding/image generation/translation/transcription/etc. There are a lot of open source models out there that anyone can retrain for more specific tasks. And we need to be careful because these larger corporations are trying to stifle that kind of competition with their lobbying efforts.
Edit: Ok it really doesn’t help when you edit your comment to provide clarification on something based on my reply as well as including additional remarks.
I mean, that’s kind of the whole point of why I was trying to nail down what the other user meant when they said “AI doesn’t provide much benefit yet”.
The definition of “AI” today is way too broad for anyone to make statements like that now.
And to make sure I understand your question, are you asking me to provide you with the definition of “AI”? Or are you asking for the definition of “AGI”?
Do bosses from video games count?
Count under the broad definition of “AI”? Yes, when we talk about bosses from video games we talk about “AI” for NPCs. And no, this should not be lumped in with any machine learning models unless the game devs created a model for controlling that NPCs behaviour.
In either case our current NPC AI logic should not be classified as AGI by any means (which should be implied since this does not exist as far as we know).
I think you’re confusing “AI” with “AGI”.
“AI” doesn’t mean what it used to and if you use it today it encompasses a very wide range of tech including machine learning models:
Speech to text (STT), text to speech (TTS), Generative AI for text (LLMs), images (Midjourney/Stable Diffusion), audio (Suno). Upscaling, Computer Vision (object detection, etc).
But since you’re looking for AGI there’s nothing specific to really point at since this doesn’t exist.
Edit: typo
I’m going to assume that when you say “AI” you’re referring to LLMs like chatGPT. Otherwise I can easily point to tons of benefits that AI models provide to a wide variety of industries (and that are already in use today).
Even then, if we restrict your statement to LLMs, who are you to say that I can’t use an LLM as a dungeon master for a quick round of DnD? That has about as much purpose as gaming does, therefore it’s providing a real benefit for people in that aspect.
Beyond gaming, LLMs can also be used for brainstorming ideas, summarizing documents, and even for help with generating code in every programming language. There are very real benefits here and they are already being used in this way.
And as far as resources are concerned, there are newer models being released all the time that are better and more efficient than the last. Most recently we had Llama 3 released (just last month), so I’m not sure how you’re jumping to conclusions that we’ve hit some sort of limit in terms of efficiency with resources required to run these models (and that’s also ignoring the advances being made at a hardware level).
Because of Llama 3, we’re essentially able to have something like our own personal GLaDOS right now: https://www.reddit.com/r/LocalLLaMA/comments/1csnexs/local_glados_now_running_on_windows_11_rtx_2060/
The first thing I said was, “the more you compress something, the more processing power you’re going to need [to decompress it]”
I’m not removing the most computationally expensive part by any means and you are misunderstanding the process if you think that.
That’s why I specified:
The drawback is that you need a powerful computer and a lot of energy to regenerate those images, which brings us back to the problem of making this data conveyed in real-time while using low-power.
And again
But of course, that’s still going to take time to decompress as well as a decent spike in power consumption for about 30-60+ seconds (depending on hardware)
Those 30-60+ second estimates are based on someone using an RTX 4090, the top end Consumer grade GPU of today. They could speed up the process by having multiple GPUs or even enterprise grade equipment, but that’s why I mentioned that this depends on hardware.
So, yes, this very specific example is not practical for Neuralink (I even said as much in my original example), but this example still works very well for explaining a method that can allow you a compression rate of over 20,000x.
Yes you need power, energy, and time to generate the original image, and yes you need power, energy, and time to regenerate it on a different computer. But to transmit the information needed to regenerate that image you only need to convey a tiny message.
This article may as well be trying to argue that we’re wasting resources by using “cloud gaming” or even by gaming on your own, PC.
Sure, but this is just a more visual example of how compression using an ML model can work.
The time you spend reworking the prompt, or tweaking the steps/cfg/etc. is outside of the scope of this example.
And if we’re really talking about creating a good pic it helps to use tools like control net/inpainting/etc… which could still be communicated to the receiving machine, but then you’re starting to lose out on some of the compression by a factor of about 1KB for every additional additional time you need to run the model to get the correct picture.
A job interview! (I wish I was joking).
The reward for developing this miraculous leap forward in technology? A job interview, according to Neuralink employee Bliss Chapman. There is no mention of monetary compensation on the web page.
You also have to keep in mind that, the more you compress something, the more processing power you’re going to need.
Whatever compression algorithm that is proposed will also need to be able to handle the data in real-time and at low-power.
But you are correct that compression beyond 200x is absolutely achievable.
A more visual example of compression could be something like one of the Stable Diffusion AI/ML models. The model may only be a few Gigabytes, but you could generate an insane amount of images that go well beyond that initial model size. And as long as someone else is using the same model/input/seed they can also generate the exact same image as someone else. So instead of having to transmit the entire 4k image itself, you just have to tell them the prompt, along with a few variables (the seed, the CFG Scale, the # of steps, etc) and they can generate the entire 4k image on their own machine that looks exactly the same as the one you generated on your machine.
So basically, for only a few bits about a kilobyte, you can get 20+MB worth of data transmitted in this way. The drawback is that you need a powerful computer and a lot of energy to regenerate those images, which brings us back to the problem of making this data conveyed in real-time while using low-power.
Edit:
For transmitting the information to generate that image, you would need about 1KB to allow for 1k characters in the prompt (if you really even need that),
then about 2 bytes for the height,
2 for the width,
8 bytes for the seed,
less than a byte for the CFG and the Steps (but we’ll just round up to 2 bytes).
Then, you would want something better than just a parity bit for ensuring the message is transmitted correctly, so let’s throw on a 32 or 64 byte hash at the end…
That still only puts us a little over 1KB (1078Bytes)…
So for generating a 4k image (.PNG file) we get ~24MB worth of lossless decompression.
That’s 24,000,000 Bytes which gives us roughly a compression of about 20,000x
But of course, that’s still going to take time to decompress as well as a decent spike in power consumption for about 30-60+ seconds (depending on hardware) which is far from anything “real-time”.
Of course you could also be generating 8k images instead of 4k images… I’m not really stressing this idea to it’s full potential by any means.
So in the end you get compression at a factor of more than 20,000x for using a method like this, but it won’t be for low power or anywhere near “real-time”.
NAND - one of the 2 you listed, or they give up.
What is this a reference to?
I thought it was the Russians that sent a dog on a one way ticket to Space?